Edge AI: The Next Generation of Artificial Intelligence for AIoT applications
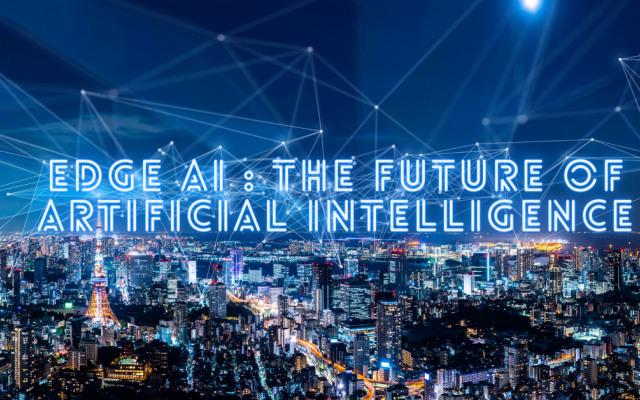
The rapid technological change has brought us closer to a world of edge AI. But what is edge AI? Edge AI combines the different levels of computing solutions and technology, where its main goal is to store, process, and manage data directly at the endpoints for machine learning and intelligence. Eventually, the rise of edge AI will significantly impact the digitalization of data enterprises focused in industrial applications. This blog will help you understand what edge AI is and its direct impact on the industrial AIoT.
Learn More About AIoT and the Future of Industrial 4.0
What is Edge AI?
Edge AI is the consolidation of edge computing and artificial intelligence (AI). By simple definition, edge computing systems gather and process data locally without being dependent on the cloud for its resources. It runs machine-learning algorithms to efficiently collect, store and process data, enabling real-time decisions within just a few milliseconds. The ability for millisecond processing gives way to an array of edge computing applications that will benefit from lower latency. Therefore, edge AI refers to Artificial Intelligence algorithms running advanced analytics for real-time processing and automation for insights. By no means will Edge AI trump the cloud in terms of its deep learning capabilities but will provide a new wave of machine intelligence right at the source. Bottom line, edge AI enables real-time decision-making for mission-critical applications, a key step to shifting industrial deployments to full-scale automation.
Edge AI Background – The migration from cloud to the edge
The evolution of AI takes root in massive data centers where exponential amounts of data are fed to AI algorithms for deep learning; this is how machine learning models are trained for intelligence. Today, Most AI applications still run in the cloud for their massive resources; But with the advent of edge computing, many machine learning models are being pushed out closer to data generation for inferencing and detection. AI that runs in the cloud can handle several simple AI applications such as voice assistance and recognition. However, various industries require more sophisticated AI solutions to manage their IoT devices at the edge. Moreover, the proliferation of IoT devices and the increase in demand for real-time computing has initiated an AI migration from the cloud to the edge.
Running AI applications at the cloud pose higher downtime risks for time-sensitive applications where bandwidth and latency are mission-critical. However, thanks to a new generation of microprocessors, edge AI algorithms can run with speed, accuracy, and efficiency. Edge AI utilizes trained Machine Learning models at the edge to alleviate data latency and bandwidth requirements. In addition, Edge AI computing allows various industries to improve their efficiencies by reducing human interventions through automation. Overall, Edge AI has impacted the industrial edge with its ability to enable real-time data processing and low-latency decision-making.
Learn More About AIoT and the Migration of AI from the Cloud to the Edge
Edge AI vs. Cloud AI Computing Architectures

Cloud computing operates by acting as a hub or repository from incoming data, usually from IoT sensors and devices. Once the data is stored in the cloud, this is where machine learning algorithms run their deep learning models for intelligence. But one disadvantage of the cloud is its physical proximity to where IoT sensors are usually deployed; this can be in harsh remote and mobile settings where resources are not as abundant as the cloud. Although the cloud has proven to be beneficial, there are some obstacles when it comes down to real-time inference and decision making. First, raw data from the sensors at the edge requires high internet bandwidth for edge to cloud telemetry. Second, not to mention the total data throughput from the millions of edge devices that pass data back to the cloud. Furthermore, edge AI use cases may include a remote environment with unstable internet connectivity where the localization of computing is a requirement for speed and accuracy.
Edge ai computing and its shift away from the cloud to deliver better bandwidth and lower-latency
In summary, edge AI is much more favorable for applications that require real-time and low-latency compute resources. Performing AI at the edge allows all-in-one, real-time operations, including decision-making, data creation, and data storage within the same device. Edge AI shifts AI models from the cloud and closer to the edge by using an inferencing model. The process for machine learning and intelligence is quite resource-heavy that requires the resources of the cloud; Deep learning algorithms are fed data and ultimately train Deep Neural Network (DNN). Once the framework for a DNN is established, it can be deployed at the edge for inference. In other words, once the computer collects all data of an object from the sensors, it utilizes all its data inputs to make predictions that are accurate. Deploying a deep learning algorithm locally at the edge enables real-time analysis with reduced latencies up to a few milliseconds. Additionally, edge AI models decrease internet bandwidth requirements, leading to lower costs from transferring data over to the cloud and back. Further below, we are going to discuss in more detail some of the benefits of edge AI computing.
Learn More About The AI Network Architectures and AI Technologies
Benefits of Edge AI

Low Latency
Information security and privacy
Edge AI frameworks are much more secure and private because they store, process, and analyze data locally at the edge instead of constantly transferring and exchanging data on the cloud. By handling all the data locally, there is a lower risk for external parties to access sensitive information due to data being processed locally as opposed to the cloud. Therefore, edge system architectures are much more private and secure, which provide an additional prevention layer against data breaches and cyber threats.
Minimal Bandwidth and Lower Cost
Without edge AI, various devices need to transfer data to the cloud continuously. As a result, there is a bandwidth bottleneck for the devices when they require the cloud for additional processing; this extra step for data transmission to and from the cloud creates bandwidth issues that can be costly. But with edge AI, there is a lower bandwidth consumption because data can be managed, stored, and processed locally, all in one device. In addition, local data analysis enhances data processing speed, which allows real-time responses.
Reliability
Finally, edge AI also benefits edge applications with much more reliability, especially for mission-critical operations. For example, autonomous vehicles and hyper-automation reap the full benefits of edge AI for its blazing-fast connections and real-time decision-making. Instead of relying on the cloud, edge AI prevents unnecessary latencies that may lead to better risk management when it matters most. In addition, edge AI can benefit by utilizing ruggedized industrial computers specifically built to withstand a wide range of harsh operating environments with their robust and durable designs, increasing its reliability to operate in outdoor industrial applications. Ruggedization requirements become extremely important when it comes to edge AI applications that are running in harsh environmental settings; these key characteristics help ensure long-term reliability.
Edge AI trends
5G
5G has been tremendously growing and is known for its ability to provide enhanced mobile broadband (eMBB), ultra-low latency communication (uRLLC), and massive Machine-Type Communications (mMTC). A key element from 5G that will help shape more edge ai applications is its mmWave spectrums that enable low-latency <1ms with 99.999% reliability. The high-speed 5G wireless connection speed will allow portable edge computers to perform AI much faster by eliminating the bandwidth bottlenecks that traditional cloud systems previously had. This trend will enable edge ai in real-world applications, where a myriad of IIoT devices can run complex AI models right at the location where the data is generated.
Learn more about 5G and The New Horizons of the Connected World
Efficient Machine Learning Models
Throughout diverse, extensive research and developments around the AI world, machine learning algorithms are getting much more efficient and accurate. More efficient machine learning models allow AI to be applied with much less computing power. Several methods such as pruning, weight sharing, quantization, and Winograd transformation have enabled low-powered AI models to run efficiently while maintaining their predictions’ accuracy. With efficient machine learning models, Edge AI can perform various complex AI models at the edge with a much lower computing power than running them in the cloud.
Learn more about Deep Learning Embedded System
Increasing Amounts of IoT-generated data
The exponential increase of IoT devices is pushing the limits of the internet bandwidth when data needs to be transferred to the cloud. To overcome the bandwidth bottleneck, Edge AI manages data locally within the device and only sends essential data to the cloud for further analysis, resulting in a faster flow of data, high performance, and increased reliability.
Industrial Demands
To stay competitive in the overgrowing industrial solutions, companies adopt various disruptive technologies, including edge AI to increase their output while reducing the Total Cost of Ownership (TCO). For example, industrial automation can dramatically increase the overall efficiency of assembly lines by implementing machine vision. Manufactures can utilize edge computers that are connected to high-speed cameras and infrared sensors to introduce automation to the assembly line. With machine vision, manufacturers can quickly detect defects from thousands of products. In addition, these edge computers are equipped with powerful AI accelerators such as GPUs and VPUs to provide real-time machine vision at the edge. As a result, more industrial applications are adopting edge AI to maintain their competitive edge.
Learn More About the Difference Between CPU vs. GPU vs. TPU
How to Choose the Best Hardware for Industrial Edge AI Computing?
Deep-learning computations require robust AI accelerators for AI systems to perform complex pattern recognition, such as intelligent surveillance or autonomous driving. Furthermore, edge AI systems will need to handle various devices and sensors that collect an enormous amount of data at the edge for the computers to process. Therefore, AI edge computers require powerful CPUs, GPUs, and NVME SSDs, to enable high-performance operations with high-capacity storage. C&T’s rugged edge computers are built with the latest technology in computing storage and connectivity in order to enable edge ai models to run efficiently.
Learn more about the Industrial PC with NVIDIA GPU
When choosing suitable Edge AI computers, the deployment environment is just as important as the hardware specification. Ruggedization features such as wide operating temperature, ingress protection, shock and vibration resistance, and even wide power input are all major requirements to consider when selecting edge ai computer. As a result, C&T has developed ultra-ruggedized edge AI computers that are suitable for industrial applications in outdoor or harsh environments such as city surveillance, mining, or robot applications.
In summary, C&T's rugged edge computers can be used as building blocks for edge AI applications that are looking to be deployed in the harshest environments that require performance and reliability. Moreover, C&T's rugged edge AI computers also utilize the best industrial-grade designs such as fanless architecture, industrial-grade materials, and cable-less designs, allowing the computers to maintain ultimate reliability.
AI Applications at the Industrial Edge
There are multiple Edge AI technologies that have been put into action at the industrial edge. For example, facial recognition, autonomous driving, and predictive maintenance are implemented in various industrial applications. To understand more about the impact of Edge AI towards the Industrial Edge, let's observe how edge AI enhancements affect some current and future industrial applications:
Smart city
Deep learning allows edge AI computers to be aware of the situations in traffic and detect abnormal behaviors through smart cameras on a real-time basis. Another smart city application that utilizes machine inferencing is license plate recognition on the road and in parking lots t AI implementation within various IoT devices allows cities to access actionable and valuable insights through edge AI. Smart cities can analyze massive amounts of data in real-time and perform actions that will help improve the city's operations for better productivity and efficiency.
Automotive
Edge AI offers control and autonomy for the automotive industry. For example, integrating edge AI into the fleet telematics industry makes it easier for users to remotely monitor the car via portable devices like phones, laptops, and tablets. Moreover, fleet vehicles companies can implement edge AI in every vehicle to provide better telematics with more control, insight, and security. Some of the available features of integrating edge AI into Fleet Vehicles are more efficient map rerouting to save gas during a trip. Also, edge AI offers automatic alerts for drivers and control sites when the system detects an abnormality that may cause downtime. In addition, since most of the computing is done locally at the edge, the fleet vehicles can process the data in real-time with lower bandwidth and power. Edge AI independence from the cloud also prevents computers from a data breach, thus increasing safety and security. In the future, edge AI will enable self-driving vehicles to reach full autonomy to drive themselves, even in rural and remote areas.
Oil and Gas
Edge AI improves the use of predictive maintenance in the oil and gas industry. It enables real-time data analysis to monitor whether or not the operation is going smoothly. For example, if the sensor detects leakage in the pipeline, the computer will command to stop the flow and alert the maintenance team. As a result, it improves the safety level of the workers and the surrounding environments. Edge AI also helps reduce costs by minimizing bandwidth requirements and downtime by moving AI computing from the cloud closer to the rugged edge computers that are located in the oil and gas asset.
Agriculture
In the agriculture industry, edge AI helps farmers keep track of the soil and weather conditions in real-time. After gathering all the data from the sensors, the edge computer can decide on the right schedule to fertilize and harvest. Edge AI also enables the machine vision to recognize and differentiate plants to select which actions to take for each plant species. With the help of edge AI, the time taken for daily management and daily harvest are reduced while increasing the harvest throughput.
Manufacturing
AI enables rugged edge computers to quickly detect defects and errors in the production chain with high-precision and real-time machine vision that human eyes cannot spot. The support of a rugged edge computer enables the process to work efficiently and reliably while maintaining high precision and lower production costs. In addition, the real-time detection analysis also allows the computer to filter out damaged goods on the production line without shutting down the machines, which will reduce downtime costs.

Why Edge AI?
Edge AI improves various intelligent applications, especially in industrial environments. Edge AI enables users to obtain high-speed data analysis and high-performance computing without relying on the cloud. Hence, choosing suitable rugged edge computers for industrial operations come as the first place to determine the success of industrial edge AI applications. Users have to understand the specific requirements for rugged edge computers that are most suitable for running AI at the industrial edge. Here C&T provides you the edge AI computers that meet industrial computing requirements that lead to deployment success. "contact us" If you have any questions about rugged edge computers for edge AI.